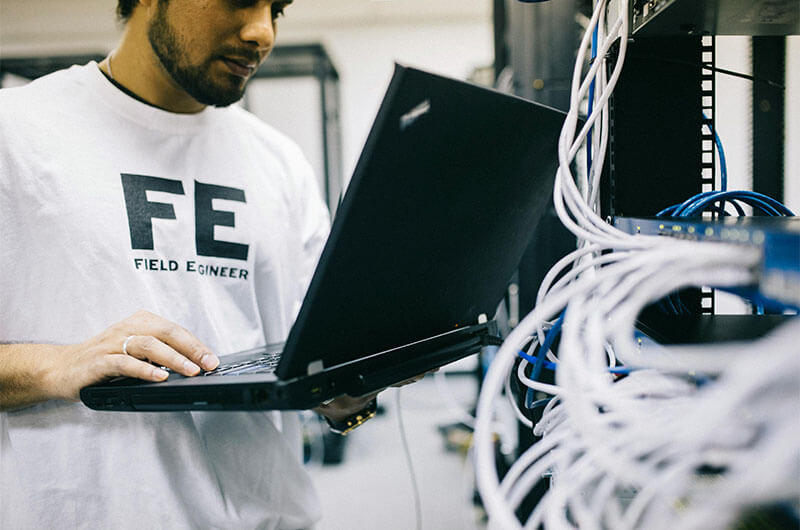
Key Takeaways:
- IoT predictive maintenance is a game-changer across industries: It’s not just for manufacturing anymore. Healthcare, automotive, and renewable energy sectors are seeing major benefits in equipment uptime, cost savings, and improved performance.
- Sensor technology is the backbone of IoT predictive maintenance: The right combination of temperature, vibration, acoustic, and other types of sensors provides crucial data needed for accurate failure predictions and proactive maintenance.
- Machine learning algorithms unlock the power of IoT data: Analyzing massive amounts of sensor data is only possible with sophisticated algorithms. These tools can identify patterns, predict failures, and even suggest the optimal timing for maintenance actions.
In This Article:
- What Is IoT Predictive Maintenance?
- How Does IoT Work in Predictive Maintenance?
- IoT and Predictive Maintenance Software
- What Algorithms Are Used in IoT Predictive Maintenance?
- Asset Management Software in Use by Our Customers
- Key Steps to Implement IoT Preventive Maintenance?
- Industrial IoT Predictive Maintenance: How to Extend Equipment Life
- What Are the Challenges of Using Internet of Things Predictive Maintenance?
- Frequently Asked Questions About IoT and Predictive Maintenance
What Is IoT Predictive Maintenance?
IOT predictive maintenance uses data collected from sensors and other IoT devices to predict when equipment might experience downtime. This enables proactive maintenance, which helps reduce the risk of downtime. It is a combination of the Internet of Things (IoT) and advanced analytics, and machine learning.
IoT predictive maintenance benefits a few industries, such as manufacturing, life sciences, and oil and gas.
The key components of IoT predictive maintenance include collecting data on equipment performance, temperature, and other parameters. It also sends data to the cloud using sensors, machine learning and advanced analytics to read data, identify patterns and anomalies, process large amounts of data, and provide maintenance teams with real-time insights that allow for predictive maintenance.
How Does IoT Work in Predictive Maintenance?
IoT devices work by collecting and transferring real-time information about equipment and machinery. This data is used to determine if the items are about to encounter problems and, thus, provide proactive maintenance to reduce downtime. IoT collects information such as temperature, vibration, pressure, usage patterns, and sensor data. The information is then passed to a centralized server or cloud, where machine learning and predictive models are used to analyze the information. The algorithms determine and identify anomalies, which then allow prediction of threats or equipment failure.
IoT provides efficient scheduling of maintenance tasks, which helps reduce downtime. It analyzes real-time data so that maintenance teams identify failures before they happen, optimize maintenance routines, and schedule maintenance during optimal times.
Over 600 Companies, Schools and Cities Rely on Timly
(No credit card required)
IoT and Predictive Maintenance Software
Using IoT for preventive maintenance has changed how industries handle hardware. Data analytics and IoT sensors help businesses predict when their equipment is going to fail and take the necessary steps to prevent downtime. Let us look at some of the top-notch software on the market to make the best use of IoT and predictive maintenance:
- SAP IoT uses machine learning and IoT to predict equipment failures and find enablers. This ensures businesses take the necessary steps to prevent downtime.
- Microsoft Azure IoT is a cloud-based system that uses machine learning and IoT to predict equipment failures and thus prevent downtime.
- Timly offers GPS trackers that provide data for proactive maintenance planning. Together with other important asset tracking software features, it optimizes equipment use by collecting and analyzing data on asset usage, maintenance history, and performance.
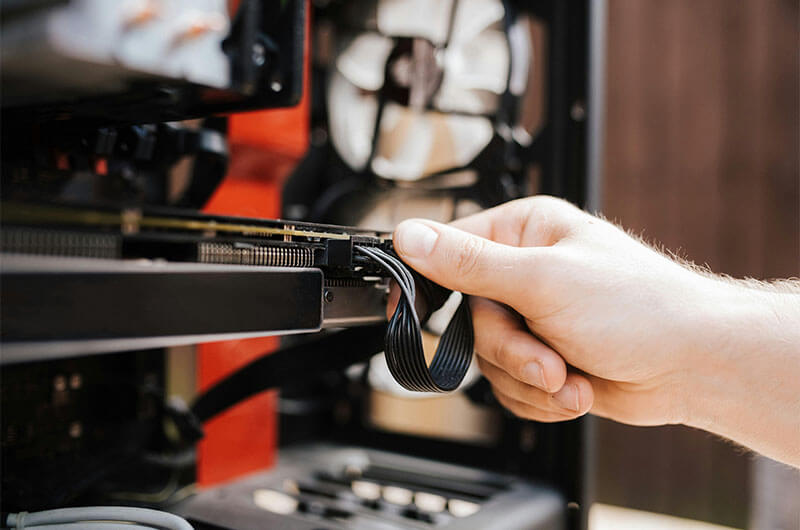
What Sensors Are Required for IoT Based Predictive Maintenance?
Choosing the righ sensors is crucial for accurate and timely predictions in predictive maintenance. Let’s explore some of the technologies and sensors used in this field:
- Temperature sensors, are used to measure fluctuations in temperatures.
- Vibration sensors help to determine the change in the vibration pattern, which can provide information on an asset’s wear and tear.
- Acoustic sensors, provides information about the sound waves that the machinery emits.
- Pressure sensors that monitor pressure changes in an asset.
- Ultrasonic sensors that use high-frequency to detect changes in equipment performance.
- Infrared sensors provide reading on temperature changes in equipment.
- Optical sensors monitors changes in the equipment’s performance.
- RFID sensors tracks equipment movement, status and location.
The right sensors for IoT-based predictive maintenance provide data accuracy, potential failure detection, lesser equipment downtime, improved asset management lifecycle phase, and overall operational efficiency.
What Algorithms Are Used in IoT Predictive Maintenance?
IoT predictive maintenance uses machine learning algorithms. It studies data from sensors and equipment and then runs predictive analysis to determine potential threats and failures. Let’s examine the integration process that utilizes machine learning algorithms to collect sensor data from equipment, cleanse and preprocess the data (removing noise), extract relevant features, train machine learning models using both labeled and unlabeled data, and ultimately monitor equipment performance.
The types of algorithms used are linear regression which predicts temperature or pressure; decision tree that reads if an equipment is going to fail; k-means clustering that identifies patterns in sensor data; and convolutional neural networks (CNNs) that analyze image and sensor data.
Advantages of Machine Learning in Predictive Maintenance Using IoT
Machine learning in predictive maintenance, combined with IoT technologies, offers significant advantages in identifying faults and preventing major failures. The benefits of using machine learning for predictive maintenance include analyzing large amounts of data, detecting anomalies and predicting failures, scheduling maintenance during planned downtime, allowing maintenance teams to respond quickly to issues before they become major problems, improving overall productivity, and reducing the risk of loss.
Asset Management Software in Use by Our Customers
The Timly software is continuously evolving to meet the needs of our customers. In various success stories, we show you how Timly optimizes processes in companies, thereby saving significant effort. With Timly, inventory management becomes child’s play.
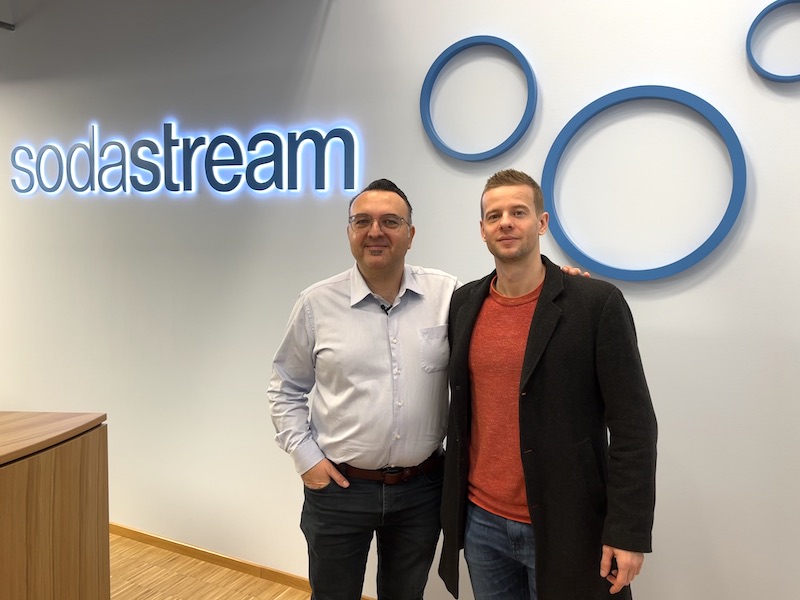
Optimized Device Management With Innovative Self-Inventory
SodaStream is the world market leader for water sparkling systems for domestic use and has a lot of IT equipment at its various locations. Many colleagues now work from their home offices. A digital solution for the efficient management of IT end devices became necessary...
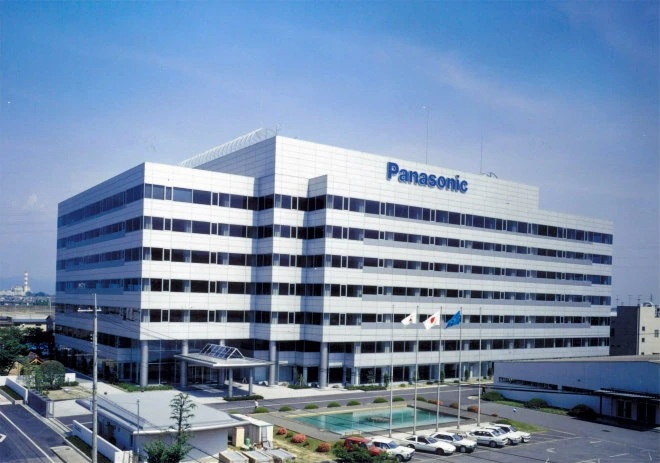
Panasonic x Timly: Driving Technological Innovation
One of the most remarkable aspects of human ingenuity is our ability to innovate. Innovation is embedded in the DNA of consumer electronics giant Panasonic, which has diversified into a number of sectors, from heavy industry to construction...
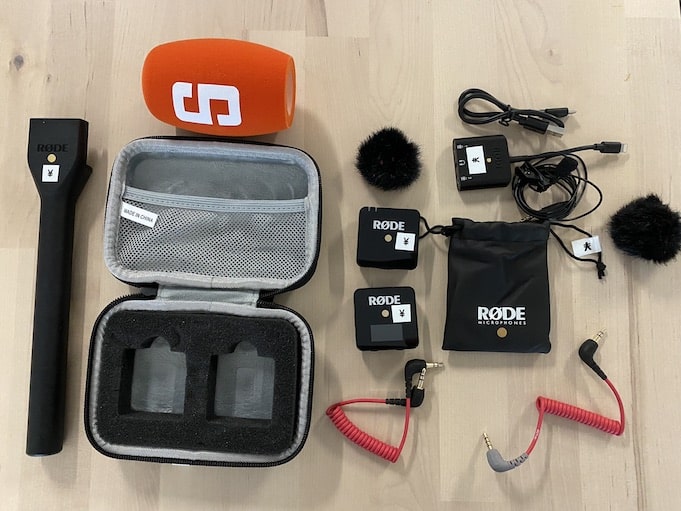
Manage Video Equipment Efficiently Without Much Effort
The Hamburg media company always does outstanding journalistic work and is characterized by independent reporting. In order to maintain journalistic quality, the teams work with highly specialized devices – these need to be managed efficiently...
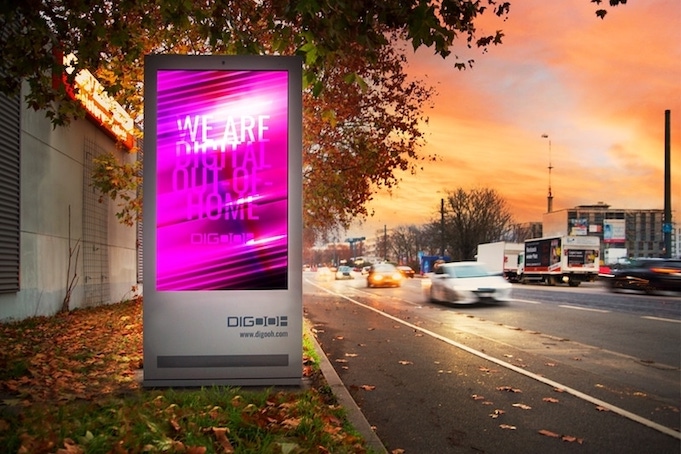
Smart City Asset Management – Timly in Use at DIGOOH
The core business of DIGOOH Media GmbH in Cologne is to manage digital city light posters (DCLP) for outdoor use in various cities in Germany. The challenge here lies in making the client’s communication message always available at the right time, in the right place...
(No credit card required)
Key Steps to Implement IoT Preventive Maintenance?
Here are the steps involved in planning and deploying an IoT predictive maintenance system:
- Define the value proposition of IoT predictive maintenance
- Determine which assets are critical to the business
- Come up with a plan to implement IoT predictive maintenance
- Determine data collection methods
To choose the right technology and partner for successful implementation, you will need to select the most suitable IoT device, sensors, and software. Moreover, it is crucial to select the right vendor, and IoT platform that can integrate with existing systems.
The best practices for integrating IoT with existing systems include integrating them with systems such as ERP, CRM, and CMMS; using APIs and SDKs; and implementing data analytics and machine learning.
To address common security concerns and mitigate them, you will need to identify potential risks and vulnerabilities in the IoT system, implement security measures such as firewalls and encryptions, and continuously monitor and maintain the security of the IoT system.
Industrial IoT Predictive Maintenance: How to Extend Equipment Life
These are the industries that use predictive maintenance and their typical use cases:
- Manufacturing: To monitor equipment performance, detect anomalies, and schedule maintenance.
- Healthcare: To monitor medical equipment, detects potential failures, and schedules maintenance repairs.
- Automotive: To monitor vehicle performance, detect potential issues, and schedule maintenance repairs.
- Renewable Energy: To monitor wind turbines, solar panels, and other equipment.
Manufacturing
In manufacturing, IoT predictive maintenance is transforming real-time monitoring and analysis of equipment performance. This helps reduce downtime and increase overall efficiency. Its benefits include reduced downtime, increased efficiency, extended equipment lifespan, and the ability for manufacturers to identify and address quality issues that could affect the final product.
Healthcare
Within the healthcare industry, IoT predictive maintenance allows real-time monitoring and helps reduce equipment downtime and improve patient care. The benefits of using IoT predictive maintenance in healthcare include reduced downtime on medical machinery, which reducesthe need for costly repairs. It also enhances data analytics that helps healthcare providers optimize maintenance schedules.
Automotive Sector
The automotive sector is utilizing real-time monitoring and analysis to enhance automation. This approach provides avenues for improved vehicle reliability, reduced downtime, and improved overall efficiency, while identifying potential issues, and enhancing the driving experience and vehicle performance.
Renewable Energy
IoT predictive maintenance is changing how renewables work by enabling real-time monitoring. This allows for proactive maintenance, reducing downtime and improving overall efficiency. Some of the benefits of IoT predictive maintenance include increased energy output, reduced downtime, cost savings through the prevention of equipment failures, and enhanced grid stability by providing optimized maintenance.
What Are the Challenges of Using Internet of Things Predictive Maintenance?
The internet of things is not without challenges and shortcomings in predictive maintenance. For one, it provides huge amounts of data, which is empowering but may also be quite overwhelming. The issue with device heterogeneity raises questions about device compatibility. Finally, sensor accuracy and reliability, as well as network connectivity and security, are far from perfect at this point in time.
To overcome these challenges, it is important to implement a robust data management strategy, standardize device communication protocols, select high-quality sensors for specific applications, and implement robust network security measures.
To continually improve and adapt IoT predictive maintenance strategies, it is crucial to monitor and analyze data, implement an interactive approach to maintenance, stay informed about new technologies, and collaborate with experts on IoT about predictive maintenance and any relevant fields.
Frequently Asked Questions About IoT and Predictive Maintenance
What Is IoT Predictive Maintenance?
What Sensors Are Required for IoT Predictive Maintenance?
In IoT Predictive Maintenance, choosing the right sensors is crucial for ensuring accurate and timely predictive data. The technologies and sensors used for predictive maintenance include:
- Temperature sensors, which are used to measure fluctuations in temperatures.
- Vibration sensors help to determine the change in the vibration pattern, which can inform an asset’s wear and tear.
- Acoustic sensors, which assist with sound waves that the machinery emits.
- Pressure sensors that monitor pressure changes in an asset.
- Ultrasonic sensors that use high-frequency to detect changes in equipment performance.
- Infrared sensors that help to read temperature changes in equipment.
- Optical sensors that help read changes in the equipment’s performance.
- RFID sensors that track equipment movement, status and location.
Recommended for You: